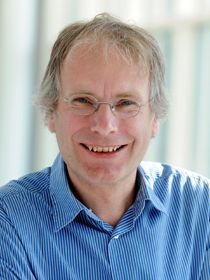
Univ.-Doz.Dipl.-Ing.Dr. Thomas Schrefl
-
thomas.schrefl@donau-uni.ac.at
-
+43 2622 23420-20
-
+43 2622 23420-99 (Fax)
-
Zum Kontaktformular
-
TFZ Wiener Neustadt, Bauteil E - Stock 2
- Universität für Weiterbildung Krems
- Zentrum für Modellierung und Simulation
- Viktor Kaplan Straße 2 - Bauteil E
- 2700 Wiener Neustadt
- Österreich
Projekte (Auszug Forschungsdatenbank)
Laufende Projekte
SINGLE GRAIN REENGINEERED NDFEB PERMANENT MAGNETS
Projektzeitraum: 01.06.2024–31.05.2028
Projektverantwortung (Universität für Weiterbildung Krems): Thomas Schrefl
Fördergeber: EU
Novel rare-earth free permanent magnets
Projektzeitraum: 01.05.2024–30.04.2028
Projektverantwortung (Universität für Weiterbildung Krems): Thomas Schrefl
Fördergeber: EU
Magnetic Multiscale Modelling Suite
Projektzeitraum: 01.01.2024–31.12.2027
Projektverantwortung (Universität für Weiterbildung Krems): Thomas Schrefl
Fördergeber: EU
Magnetic energy conversion of waste heat
Projektzeitraum: 01.01.2024–31.12.2027
Projektverantwortung (Universität für Weiterbildung Krems): Thomas Schrefl
Fördergeber: EU
Multi-property Compositionally Complex Magnets for Advanced Energy Applications
Projektzeitraum: 01.06.2023–31.05.2026
Projektverantwortung (Universität für Weiterbildung Krems): Thomas Schrefl
Fördergeber: EU
Projektzeitraum: 01.04.2023–31.03.2025
Projektverantwortung (Universität für Weiterbildung Krems): Thomas Schrefl
Fördergeber: EU
Projektzeitraum: 01.07.2022–30.06.2026
Projektverantwortung (Universität für Weiterbildung Krems): Thomas Schrefl
Fördergeber: EU
Magnet design through physics informed machine learning
Projektzeitraum: 01.09.2020–31.08.2027
Projektverantwortung (Universität für Weiterbildung Krems): Thomas Schrefl
Fördergeber: Private (Stiftungen, Vereine etc.)
Projektzeitraum: 01.01.2019–31.12.2021
Projektverantwortung (Universität für Weiterbildung Krems): Thomas Schrefl
Fördergeber: FFG
Förderprogramm: Produktion der Zukunft
Abgeschlossene Projekte
Simumag - GFF Horizon Europe Anbahnungsfinanzierung
Projektzeitraum: 01.01.2022–30.03.2022
Projektverantwortung (Universität für Weiterbildung Krems): Thomas Schrefl
Fördergeber: Bundesländer (inkl. deren Stiftungen und Einrichtungen)
Projektzeitraum: 01.03.2018–28.02.2022
Projektverantwortung (Universität für Weiterbildung Krems): Thomas Schrefl
Fördergeber: EU
Förderprogramm: Horizon 2020
Nanostructured multiphase permanent magnets
Projektzeitraum: 01.04.2019–31.12.2020
Projektverantwortung (Universität für Weiterbildung Krems): Thomas Schrefl
Fördergeber: sonstige öffentlich-rechtliche Einrichtungen (Körperschaften, Stiftungen, Fonds)
Atomistic Simulation of rare-earth reduced permanent magnets
Projektzeitraum: 01.11.2017–31.12.2019
Projektverantwortung (Universität für Weiterbildung Krems): Thomas Schrefl
Fördergeber: Unternehmen
NOVel, critical materials free, high Anisotropy phases for permanent MAGnets, by design
Projektzeitraum: 01.04.2016–30.09.2019
Projektverantwortung (Universität für Weiterbildung Krems): Thomas Schrefl
Fördergeber: EU
Förderprogramm: H2020
Vicom Multiscale simulations of magnetic nanostructures
Projektzeitraum: 01.01.2015–30.06.2019
Projektverantwortung (Universität für Weiterbildung Krems): Thomas Schrefl
Fördergeber: FWF
Förderprogramm: FWF
Nanostructured multiphase permanent magnets
Projektzeitraum: 01.04.2018–31.03.2019
Projektverantwortung (Universität für Weiterbildung Krems): Thomas Schrefl
Fördergeber: Sonstige
NanoStructured Multiphase Permanent Magnets
Projektzeitraum: 01.04.2017–31.03.2018
Projektverantwortung (Universität für Weiterbildung Krems): Thomas Schrefl
Fördergeber: andere internationale Organisationen
Nano-Structured Multi-Phase Permanent Magnets II
Projektzeitraum: 01.04.2016–31.03.2017
Projektverantwortung (Universität für Weiterbildung Krems): Thomas Schrefl
Fördergeber: andere internationale Organisationen
CREST III Simulation of hard magnet magnetic materials
Projektzeitraum: 01.04.2016–31.03.2017
Projektverantwortung (Universität für Weiterbildung Krems): Thomas Schrefl
Fördergeber: andere internationale Organisationen
Förderprogramm: CREST
Publikationen (Auszug Forschungsdatenbank)
Luca, S.; Fischbacher, J.; Flament, C.; Sedek, R.; de Rango, P.; Gomez Eslava, G.; Schrefl, T. (2025). Microstructure and magnetic properties of the Cu-rich Nd(Fe,Mo)12 strip cast flakes. Journal of Alloys and Compounds, 1010: 178039
Sandoval, C.; Miguel, A.; Jurczyk, J.; Skoric, L.; Sanz-Hernández, D.; Leo, N.; Kovacs, A.; Schrefl, T.; Hierro-Rodríguez, A.; Fernández-Pacheco, A. (2025). Remote-sensing based control of 3D magnetic fields using machine learning for in operando applications. Journal of Applied Physics, Vol. 137, no 11: 113905-1 - 113905-7
Zenbaa, N.; Abert, C.; Majcen, F.; Kerber, M.; Serha, R.; Knauer, S.; Wang, Q.; Schrefl, T.; Suess, D.; Chumak, A. (2025). A universal inverse-design magnonic device. Nature Electronics, Vol. 1: 1
Ali, Q.; Fischbacher, J.; Kovacs, A.; Özelt, H.; Gusenbauer, M.; Moustafa, H.; Böhm, D.; Breth, L.; Schrefl, T. (2024). Defect manipulation for the coercivity enhancement of Nd-Fe-B permanent magnets. Physica B: Condensed Matter, Vol. 678: 415759
Brueckl, H.; Breth, L.; Fischbacher, J.; Schrefl, T.; Kuehrer, S.; Pachlhofer, J.; Schwarz, M.; Weirather, T.; Czettl, C. (2024). Machine learning based prediction of mechanical properties of WC-Co cemented carbides from magnetic data only. International Journal of Refractory Metals and Hard Materials, Vol. 121: 106665
Gusenbauer, M.; Stanciu, S.; Kovacs, A.; Oezelt, H.; Fischbacher, J.; Zhao, P.; Woodcock, T. G.; Schrefl, T.; Stanciu S. (2024). Micromagnetic study of grain junctions in MnAl-C containing intergranular inclusions. Elsevier Journal of Magnetism and Magnetic Materials, Vol. 606: 172390
Kovacs, A.; Exl, L.; Kornell, A.; Fischbacher, J.; Hovorka, M.; Gusenbauer, M.; Breth, L.; Oezelt, H.; Yano, M.; Sakuma, N.; Kinoshita, A.; Shoji, T.; Kato, A.; Schrefl, T. (2024). Image-based prediction and optimization of hysteresis properties of nanocrystalline permanent magnets using deep learning. Journal of Magnetism and Magnetic Materials, Vol. 596: 171937
Moustafa, H.; Kovacs, A.; Fischbacher, J.; Gusenbauer, M.; Ali, Q.; Breth, L.; Hong, Y.; Rigaut, W.; Devillers, T.; Dempsey, N. M.; Schrefl, T.; Özelt, H. (2024). Reduced Order Model for Hard Magnetic Films. AIP Advances, Vol. 14, iss. 2: 025001-1 bis 025001-5
de Moraes, I. G.; Fischbacher, J.; Hong, Y.; Naud, C.; Okuno, H.; Masseboeuf, A.; Devillers, T.; Schrefl, T.; Dempsey, N. M. (2024). Nanofabrication, characterisation and modelling of soft-in-hard FeCo–FePt magnetic nanocomposites. Acta Materialia, Vol. 274: 119970
Breth, L.; Fischbacher, J.; Kovacs, A.; Özelt, H.; Schrefl, T.; Brückl, H.; Czettl, C.; Kührer, S.; Pachlhofer, J., Schwarz, M. (2023). FORC diagram features of Co particles due to reversal by domain nucleation. Journal of Magnetism and Magnetic Materials 571 (2023) 170567 Available online 24 February 2023 0304-8853/© 2023 Elsevier B.V. All rights reserved.Contents lists available at ScienceDirect Journal of Magnetism and Magnetic Materials, Vol. 571: 1-6
Breth, L.; Schrefl, T.; Fischbacher, J.; Oezelt, H.; Kovacs, A.; Czettl, C.; Pachlhofer, J.; Schwarz, M.; Brueckl, H. (2023). Micromagnetic simulations as a tool for bottom-up explainability of FORC diagrams. Proceedings in AIM IEEE Advances in Magnetics 2023, Vol. 1: 1
Kovacs, A.; Fischbacher, J.; Oezelt, H.; Kornell, A.; Ali, Q.; Gusenbauer, M.; Yano, M.; Sakuma, N.; Kinoshita, A.; Shoji, T.; Kato, A.; Hong, Y.; Grenier, S.; Devillers, T.; Dempsey, N. M.; Fukushima, T.; Akai, H.; Kawashima, N.; Miyake, T.; Schrefl, T. (2023). Physics-Informed Machine Learning Combining Experiment and Simulation for the Design of Neodymium-Iron-Boron Permanent Magnets with Reduced Critical-Elements Content. Frontiers in Materials 2023, Vol. 9: 1-19
Okabe, R.; Li, M.; Iwasaki, Y.; Regnault N.; Felser, C.; Shirai, M.; Kovacs, A.; Schrefl, T.; Hirohata, A. (2023). Materials Informatics for the Development and Discovery of Future Magnetic Materials. IEEE Magnetics Letters, vol. 14: 1-5
Schaffer, S.; Schrefl, T.; Oezelt, H.; Kovacs, A.; Breth, L.; Mauser, N.J.; Suess, D.; Exl, L. (2023). Physics-informed machine learning and stray field computation with application to micromagnetic energy minimization. Journal of Magnetism and Magnetic Materials, 576: 170761
Yamano, H.; Kovacs, A.; Fischbacher, J.; Danno, K.; Umetani, Y.; Shoji, T.; Schrefl, T. (2023). Efficient optimization approach for designing power device structure using machine learning. Japanese Journal of Applied Physics, Vol. 1: 1-17
Zhao, P.; Gusenbauer, M.; Oezelt, H.; Wolf, D.; Gemming, T.; Schrefl, T.; Nielsch, K.; Woodcock, T. G. (2023). Nanoscale chemical segregation to twin interfaces in t -MnAl-C and resulting effects on the magnetic properties. Journal of Materials Science & Technology, Vol. 134: 22-32
Ali, Q.; Fischbacher, J.; Kovacs, A.; Oezelt, H.; Gusenbauer, M.; Yano, M.; Sakuma, N.; Kinoshita, A.; Shoji, T.; Kato, A.; Schrefl, T. (2023). Benchmarking for systematic coarse-grained micromagnetics. In: HMM, proceedings in 13th International Symposium on Hysteresis Modeling and Micromagnetics (HMM 2023): 1, HMM, WIen
Fischbacher, J.; Schrefl, T.; Moraes, I.; Dempsey, N. (2023). Micromagnetic modelling of soft-in-hard FeCo-FePt nanocomposites. In: HMM, proceedings in 13th International Symposium on Hysteresis Modeling and Micromagnetics (HMM 2023): 1, HMM, Wien
Gusenbauer, M.; Oezelt, H.; Kovacs, A.; Fischbacher, J.; Zhao, P.; Woodcock, T.-G.; Schrefl, T. (2023). Magnetization reversal of large granular magnetic materials. In: HMM, proceedings in 13th International Symposium on Hysteresis Modeling and Micromagnetics (HMM 2023): 1, HMM, Wien
Hrushko, O.; Schrefl, T. (2023). Some approaches for estimating thermal residual stresses in a polycrystalline Nd2Fe14B magnet. In: HMM, proceedings in 13th International Symposium on Hysteresis Modeling and Micromagnetics (HMM 2023): 1, HMM, Wien
Vorträge (Auszug Forschungsdatenbank)
Micromagnetism: From basic theory to simulations
CRMMM 2024, 25.11.2024
Multiscale design of permanent magnets
The 11th International Conference on Multiscale Materials Modeling, 25.09.2024
Materials informatics for permanent magnet optimization
Frontiers in Magnetism: Magnetic Materials and Motors for Green Energy Applications, 16.09.2024
Micromagnetics and artificial intelligence for rare-earth free permanent magnet design
Workshop: Sustainable Materials for Energy Production, 19.08.2024
Inverse design of permanent magnets
32nd International Materials Research Congress, 19.08.2024
AI enhanced micromagnetics for permanent magnets
International Conference on Magnetism, 03.07.2024
Magnetic Multiscale Modelling Suite
Materials week 2024, 18.06.2024
Artificial intelligence for magnetic materials design
9th INTERNATIONAL CONFERENCE on SUPERCONDUCTIVITY and MAGNETISM, 30.04.2024
The basics of micromagnetic simulations, Applications of micromagnetism, Machine learing in magnetic materials design
9th Spring School and Educational Courses in ICSM 2024, 25.04.2024
Generative artificial intelligence for inverse design of permanent magnets
WPI & MMM workshop on "Inverse-design magnonics", 20.02.2024
MATERIALS INFORMATICS FOR PERMANENT MAGNET DESIGN
The 4th DXMaG Seminar, 06.12.2023
Materials informatics for permanent magnet design
Materials Innovation Strategy Symposium 2023, 05.12.2023
Modelling Magnets: From Atoms to Bulk Properties
Biomagnetic Sensing Seminar, 16.11.2023
Spotting the next big idea
68th Annual Conference on Magnetism and Magnetic Materials, 02.11.2023
Talking about magnets – information retrieval with large language models
68th Annual Conference on Magnetism and Magnetic Materials, 31.10.2023
The coercivity of permanent magnets: Insights from micromagnetics and machine learning
Physics of Magnetism 2023, 30.06.2023
Magnetization reversal of large granular magnetic materials
HMM 2023, 05.06.2023
Multiscaling strategies in computational magnet design
Going Green – CARE INNOVATION 2023, 11.05.2023
Generative deep learning for permanent magnet microstructures
67th Annual Conference on Magnetism and Magnetic Materials (MMM 2022), 03.11.2022
How to Create an Effective Scientific Video Presentation
67th Annual Conference on Magnetism and Magnetic Materials (MMM 2022), 02.11.2022